Hundred Plus
The condition of a structure - similar to that of people - is characterised by an ever more rapid degradation as it ages. Preventive measures against ageing are all the more successful the earlier they are taken. To prolong the usability of complex structures, much more information is needed at a much earlier stage than is usual today. To drastically reduce this deficit and move towards predictive maintenance, fundamental research is needed on the methods of collecting, linking and evaluating all data on geometry, material, stress and ageing. Digitisation, especially the concept of the digital twin, takes on a completely new significance in this context. It enables the combination and real-time evaluation of all data required for operation and maintenance. However, the construction industry is faced with special challenges in terms of content and methodology: buildings, especially in transport infrastructure, are always unique. They are characterised by enormous dimensions and have a considerably longer service life than other technical facilities. Their rate of change due to deterioration is very low and thus hardly measurable. The SPP "Hundred plus" addresses these challenges in three interdisciplinary research areas:
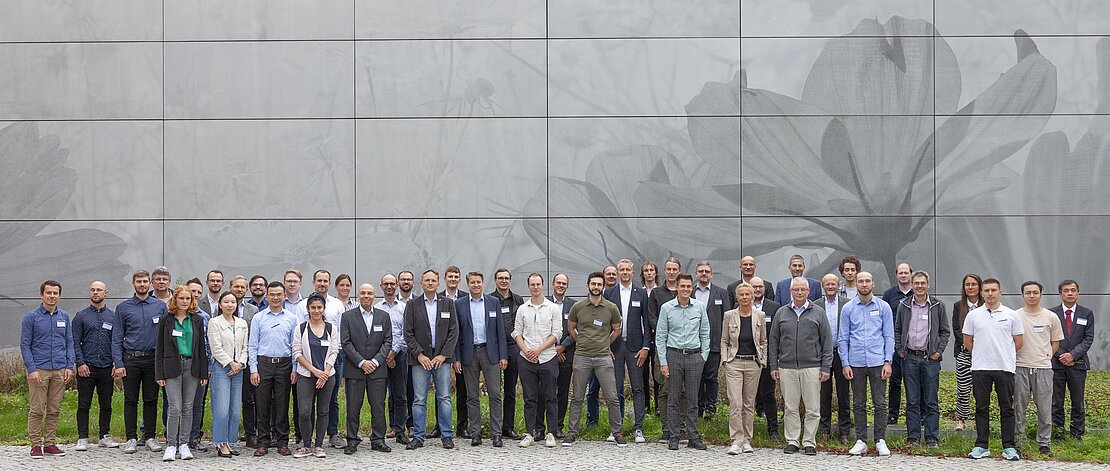
Research area (1): Digital models
The "Digital Models" department develops methods for generating largely automated, georeferenced, object-oriented 3D models from heterogeneous as-built data of engineering structures, supported by digital construction recording procedures, which contain semantic information in addition to the geometry.
No digital models exist for the majority of infrastructure structures in Germany. In addition, the as-built data is very heterogeneous and ranges from unscaled sketches to scanned plans and building information models. The information is often contradictory or incorrect. Although infrastructure managers have computer technology and structures at their disposal to organise building information digitally, the data from different project phases is often kept in different systems and only manually correlated. For road bridges, for example, databases such as the Road Information Bank for Structures (SIB-Bauwerke) are used, but these do not have a component-related object orientation and only allow limited information mapping. Further information and documents on one and the same object can only be derived from analogue hand sketches and archived planning documents.
As-built recording methods such as terrestrial laser scanning or photogrammetry can significantly improve the data situation and thus support the modelling. Drones (Unmanned Aerial Vehicle, UAV) also contribute to the automation of the condition assessment of structures.
The use of optical sensors enables high-precision (3D) structural recording of complete objects. Digital optical systems (e.g. laser scanners, videotachymeters, 3D cameras, etc.) play a decisive role in the recording and monitoring of three-dimensional geometries. Here the research focus is on distributed sensor systems for cooperative recording and the development of stationary or mobile multi-sensor systems.
3D volume models are generated from the recorded point clouds by manually tracing the cross-sections or triangulation. Based on this, an as-built BIM model with a great depth of information is created. To generate digital building models, a distinction is made between calculating methods (data description in functions) and explaining methods (data derivation from given boundary conditions). The transition from the 3D to the BIM model is made more difficult by the lack of semantic information. Parametric and associative relationships of assemblies and components can support the modelling process. The enrichment and linking of the BIM model with semantic information, further databases and documents creates the basis for the digital twin and thus for the digital maintenance management of infrastructure structures.
Therefore, in addition to the use of optical sensors to record the surface characteristics of an object, fusion with sensors that allow conclusions to be drawn about the structure of the object is also an important area of research. Self-learning algorithms (ML) or computer vision (CV) are used to process captured images. The semantic information is labelled in the images with the help of image-based artificial neural networks (ANN). Methods based on image and text recognition that enable automated derivation of information from the execution and as-built plans can also be included.
Significant research gaps in the area of "digital models" are currently evident in the automated processing of heterogeneous, analogue as-built documents and, above all, their linking with the results of digital building survey procedures. In addition to the insufficient automation, there are also no methods in which the information on the origin, accuracy and correctness of the input data is preserved.
Research area (2): Digital linkage
The focus of the area "Digital Linkage" is how the time-variant condition information of the real object can be processed in real-time and linked to the digital twin.
Today, the maintenance management of infrastructure is significantly influenced by the results of manual periodic assessments. In both road and railway bridge construction, "manual" inspections are normatively required every six years. In exceptional cases, this preventive maintenance strategy is supplemented by structural monitoring when damage occurs. However, neither the location nor the time of occurrence of damage can be predicted with this. It is also problematic for continuously measuring monitoring systems that no generally valid monitoring concepts are possible due to the large dimensions and the high individuality of the infrastructure structures. The research gap is therefore in the development of a predictive monitoring concept with initially unknown damage location and time. For the digital twin, the monitoring system and the periodic in-situ inspections are further essential components.
A current field of research is the optimal sensor placement. While relevant measuring points for static quantities are often determined with a priori models (e.g. FE models), so-called Sensor Placement Optimisation (SPO) procedures are also used for vibration-based structural monitoring. Besides metaheuristic methods, the Effective-Independence (EI) approach describes an efficient iterative algorithm to reduce a given set of sensor positions until the optimal number of sensors is reached for sufficient reliability of the measurement result. The combination of optimal sensor placement, wireless sensor networks and costs are also relevant to research.
Sound-based methods have great potential for damage monitoring when the location of the damage is initially unknown. Currently, more and more stationary sensor systems on the structure are being investigated. In describing different damage mechanisms, the developed systems aim at the exact localisation and determination of the intensity of possible damage. An important area of research is, for example, bridges with prestressing steel at risk of stress corrosion cracking. Here, metrological long-term monitoring is of enormous importance, as the early detection of individual prestressing wire breaks can prevent sudden structural failure. In addition, ultra-sonic and impact-echo methods are being investigated to locate and observe grouting faults or cavities as well as degradation phenomena.
Current research in the field of mobile sensor systems is concerned with the development of sensor applications on moving platforms (e.g. drones or robots) including orientation and positioning for highly accurate and fast object monitoring. But also conventional measurement techniques, such as terrestrial laser scanning or videotachymetry, are studied in order to detect damage to bridges more efficiently.
In addition to the sensor hardware, data management is essential for building monitoring. In particular, continuous data pre-processing (e.g. error detection, data plausibility checks and cleaning) still requires extensive basic research.
Currently, new processes for data acquisition (e.g. for evaluating data quality) are being developed. Research approaches for error diagnosis aim, for example, at an "analytical redundancy" of the Structural Health Monitoring (SHM) and model errors in the monitoring system.
The linking of information from in-situ inspections (surveys, non-destructive and destructive testing), quasi-real-time measurement data (SHM) and graphical representation (BIM) requires a new type of central platform that integrates all building information and realises data exchange with the real object. This information can be accessed by any user at any time. However, it must be researched how the data continuously collected in building monitoring can be integrated into this platform and further processed in the model. The coupled models will be examined with regard to their reliability, security and robustness. These modelling approaches, which are initially adapted to building monitoring, will be extended to include concepts of adaptivity and structural control. For the representation and adaptation of the digital twin, Industry Foundation Classes (IFC) are often used in research as a basis, which enables a comprehensive and standardised data format for the software-neutral exchange of digital building models. Current research efforts are pursuing the approach of a formal IFC-based query language with which data such as structural, building or management information can be accessed in order to describe building monitoring systems holistically and in conformity with standards using the IFC format.
Research area (3): Conditon indicators
In order to automatically convert huge amounts of data of the most varied structure and origin (Big Data) into condition information that can be quickly and largely intuitively grasped by humans, the "Condition Indicators" department is developing scientific methods for their automated derivation from continuous measurement data and from data from classic maintenance and inspection. The condition indicators and their prognosis are developed on the basis of physical models and also purely data-supported on the basis of mathematical models and with the help of artificial intelligence. Based on so-called key performance indicators (KPIs), which form an assessment standard in the planning and control of companies with the help of a key figure, condition indicators (CIs) describe an information-condensed condition of a building structure or a technical facility. They enable a quick and intuitive judgement in the condition assessment of the object. In the course of "Industry 4.0", research is being conducted on maintenance strategies that focus on predictive monitoring, especially in plant construction, aerospace and the automotive industry. The procedure first involves structuring large amounts of data. Then the analysed measurement data is used to develop self-learning algorithms (e.g. cluster analysis). This information in turn allows the calculation of the condition prognosis of the respective monitored building component. For structures, however, scientific methods for processing the relevant heterogeneous information into condition indicators are still lacking. For this purpose, measurable and quantifiable performance indicators (PIs) have to be defined in relation to bridge performance. The high number of possible PIs can be reduced by a higher-level classification through the definition of KPIs. Important KPIs are, for example, reliability, maintainability or the assessment from the building survey. They can be divided into main groups in which the individual PIs are evaluated numerically and weighted with a defined factor.
Instead of the general term Performance Indicator, the synonym condition indicator is introduced for structural condition monitoring. In this indicator, gigantic amounts of data are to be automatically summarised, processed and converted into condition information. Building on the acquisition of measurement data and the generation of information in the "Digital Linkage" FB, data and condition forecasting are essential for the "Condition Indicators" FB in addition to data analysis and aggregation. In the data analysis, the different measurement data are linked with each other and logical conclusions are drawn. Methods for assessing the automatically analysed measurement data are the basis for human decision-making. With data aggregation, the information is bundled and input variables are generated for the algorithms that are used to calculate state forecasts. The forecasts can be used, for example, to make damage predictions.
Current research is concerned with model-based and statistical approaches. While the former describes the physical system and measurement behaviour mathematically and is particularly suitable for data analysis, purely mathematical-statistical approaches such as supervised and unsupervised machine learning methods are used for data aggregation. Research is being conducted primarily with regard to the mathematical description of the physical system and measurement behaviour for state prediction.
Data-driven prognosis models are also being continuously developed. In addition to purely measurement-based forecasts generated with machine learning methods, it is primarily physical forecast models that are combined with SHM data. The latter method allows for an adaptation of the structure-specific model parameters. The prediction of building reactions in combination with as-built and monitoring data is called FE update. The adaptive FE model can be understood as an analogy to machine learning, which is furthermore interpretable and can be used for early detection of damage. Current research aims, for example, at reducing the high computational intensity and describing structure-specific signal features.